The interdisciplinarity of neuroscience: Bridges between brain, artificial intelligence and physics.
In recent years, the recognition of neuroscience as a highly interdisciplinary branch of knowledge has become more than evident. On the one hand, we have recent advances in artificial intelligence, whose foundations are based on taking inspiration from our brains to build more efficient information processing algorithms. Concepts such as learning, synaptic plasticity, receptive fields, or attention, typically associated with classical neuroscience and psychology, are now common in areas such as computer vision or ChatGPT-like language models. On the other hand, researchers are increasingly aware of the urgent need to use interdisciplinary approaches (combining, for example, disciplines such as neuroscience, complex systems physics, and artificial intelligence) to understand the brain’s functioning and mental illnesses. Several pioneering researchers in computational neuroscience – a field that makes use of theoretical and computational techniques to study how the brain works – have recently been awarded top-level prizes, such as the Brain Prize (awarded to Larry Abbot, Haim Sompolinsky, and Terry Sejnowski) or, more recently, the Nobel Prize in Physics (in the case of John Hopfield and Geoffrey Hinton).
This points to a symbiosis between mathematics and neurobiology: using mathematics to translate neuroscience concepts to different engineering problems has proven to be tremendously helpful (I refer to the examples above), but we can also use mathematics and computer science to understand better the original problem: how the brain works. This is not a new concept: virtually all scientific disciplines use computer models to understand complex phenomena better, from the motion of galaxies to volcanic eruptions or interactions between atoms. In the case of neuroscience, however, the issue becomes somewhat misleading since this is the only case where we use neural network algorithms to study not just any object but the one that initially inspired these algorithms. In other words, when we implement a simulation of neural networks in our computer, do we want to generate artificial algorithms capable of solving a concrete problem, such as evaluating the best stock market investment or identifying pedestrians crossing the street? Or is our goal perhaps to simulate the “real” neural network to understand how our brain acts when we perceive or remember something? This is a subtle but very relevant distinction: in the first case, which we can call a neuronal-network tool, the biological inspiration must give way to more practical considerations, such as maximizing the efficiency of the algorithm or evaluating the energy consumption of the algorithm. In the second case, which we will call red-neuronal-as-model, the key is to develop a model that, with a greater or lesser level of detail, focuses on improving our understanding of the physical object itself (the brain), which implies staying close to the biological aspects of it.
A clear example is the use of neural network models for clinical applications. From a more pragmatic point of view or a neural network as a tool, a researcher can program a convolutional neural network algorithm and train it on images from brain scans to identify signs of alterations in the brain linked to various diseases. In this case, we are not interested in how biologically realistic the neural network used is as long as it gives good results.

Neural networks as a model
On the other hand, from a more fundamental, or neuronal-network-like-model, point of view, a researcher can program a neural network so that neurons and synapses have a similar structure and properties to those of the brain. In the case of simulating a whole brain, our model could be used to identify the causes of certain mental illnesses – such as reduced connectivity between neurons in frontal and parietal regions in the case of schizophrenia.
Neural-networks-as-model does not necessarily require an extremely high level of detail but instead aligns the existing level of detail in the model with neurobiological evidence. For example, my lab (the Computational Neuroscience Lab at the University of Amsterdam) has recently worked with experimental collaborators to study the biological origin of the Yerkes-Dodson law. This law, which is now more than a hundred years old, is a psychological principle in which people perform tasks optimally when stress levels are moderate (i.e., not drowsy, but not highly agitated either). That is one of the reasons why, for example, we tend to do better on exams when we are well-rested and not too nervous. Although the law is over a hundred years old, its neurobiological origin is still unclear. Our study (Beerendonk, Mejias et al., PNAS 2024 ) presents a computational model that replicates the Yerkes-Dodson law and, at the same time, proposes that the underlying biological mechanism is interactions between various types of neurons in the neocortex, which combine sensory information with attentional or wakefulness signals in humans. This is an example of how simple computational models can help to understand complex psychological processes better.
Since the focus of model-like neural networks is not on maximizing the efficiency of the resulting algorithm, it is evident that these models are not as effective as tool-like neural networks in specific tasks such as image recognition. However, this does not mean they cannot perform simple tasks. In a parallel line of research, our laboratory is developing models of ‘digital brains’ that contain a considerable level of biological realism and can replicate simple cognitive tasks related to short-term memory (Mejias and Wang, eLife 2022; Feng et al. BioRxiv 2023) or decision-making ( Zou et al. BioRxiv 2024 ). These models allow us to bring together experimentally observed neural activity patterns and the basic cognitive skills of perception and memory for the first time in the same theoretical framework. Using such models will allow a better understanding of the connection between irregularities in neuronal activity and cognitive problems associated with mental disorders, a line of research with high clinical potential that we are currently developing.
Finally, neural networks as tools or models need not be mutually exclusive. Although there is currently some degree of division, or specialization, between the two branches, the ambition to combine both approaches will likely be a point of intense research shortly (van Holk and Mejias, Curr. Op. Behav. Sci. 2024). The resulting models could provide the advantages of both approaches in the same theoretical framework and allow, for example, the integration of the biological realism and high computational capacity needed for brain implants that can replace damaged brain areas. Although no complete examples of such models exist yet, we know they are plausible – after all, we have the most substantial proof on our shoulders.
Can you help us grow? Become a member and participate. Spread the word on the networks. Contact us and tell us about yourself and your project.
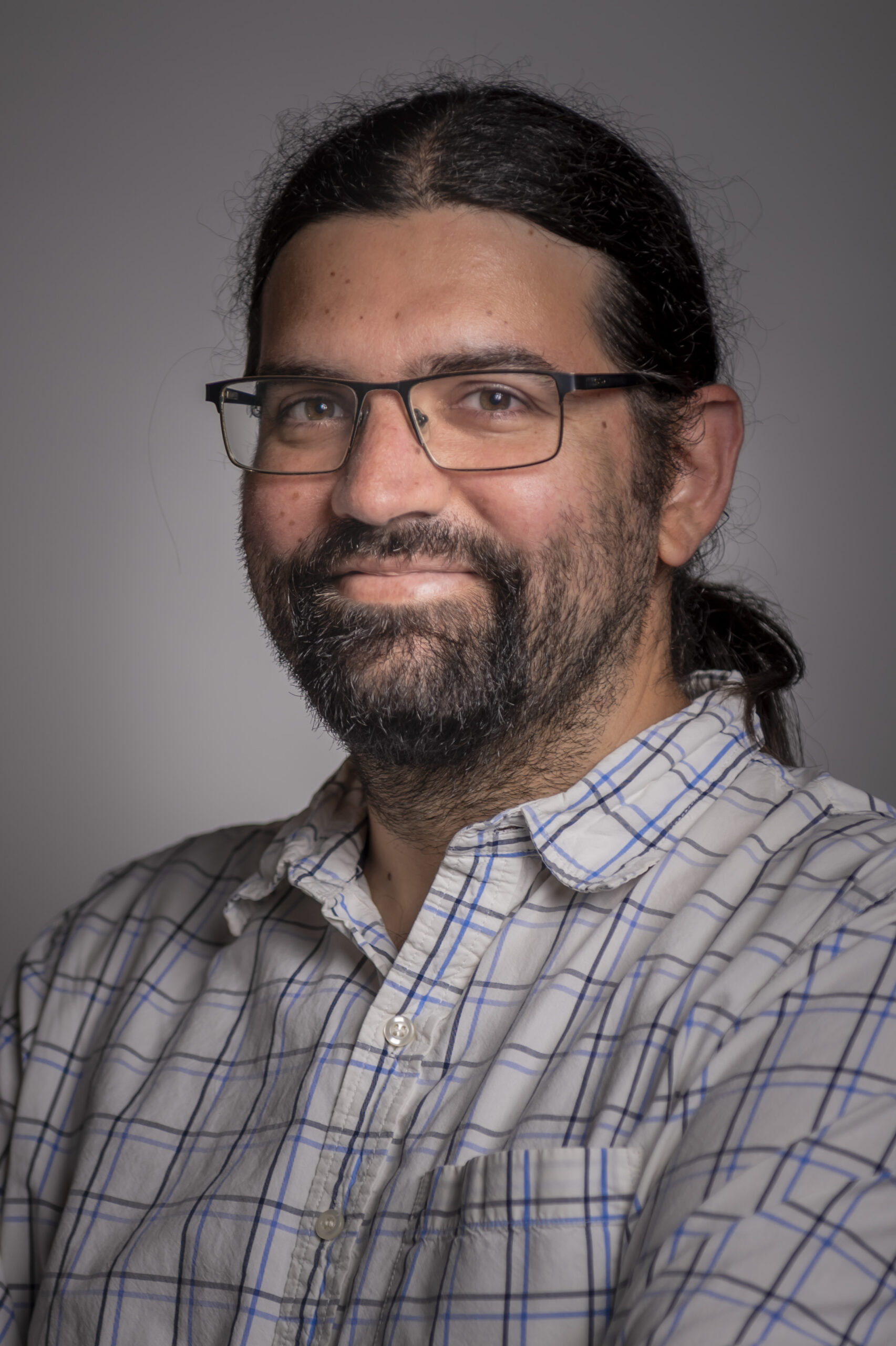
Jorge Mejías
Principal Investigator and Assistant Professor, University of Amsterdam
Born in Cáceres and raised in Cádiz, my interest in neuroscience arose during my Physics studies, where I learned to see the brain as the perfect example of a “complex system.” I specialized in the field of Computational Neuroscience during my Master’s and PhD stages at the University of Granada, and later as a postdoctoral researcher at the Universities of Ottawa (Canada), New York (USA), and NYU Shanghai (China). In 2017, I moved to the University of Amsterdam as an Assistant Professor to establish my lab and a research line focused on developing mathematical and computational models of the brain to study cognitive processes. The approach of my lab is very close to neurobiology: instead of creating artificial neural network models, computationally powerful but with little connection to biology, our computational models focus on replicating the dynamics and behavior of real neural circuits, using structural neuroanatomy and neuroimaging data. This neurobiological modeling, combined with techniques from statistical physics, complex systems, and machine learning, has allowed us to establish a series of theoretical and computational models with which to reproduce brain dynamics at multiple scales, from the activity of individual neurons to complex behaviors in animals such as memory or decision-making.